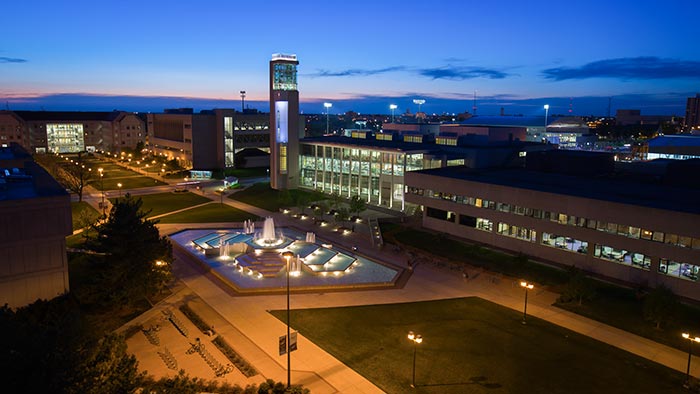
Simon Batzner
Applied Mathematics
Harvard University
Abstract:
Symmetry plays a central role in the representation of materials for the purpose of Machine Learning. In particular, all sensible representations must obey the symmetries of 3D space: translation, rotation, and inversion, in addition to permutation symmetry with respect to the labeling of atoms. Traditionally, representations have been constructed to possess invariance with respect to the above transformations. In this talk, I will discuss our efforts to generalize invariance to the broader class of equivariant representations and demonstrate how this leads to a large increase in generalization accuracy and sample-efficiency of the learned models. The talk will then discuss the recently introduced Neural Equivariant Interatomic Potential (NequIP) and Allegro potentials, two E(3)-equivariant Interatomic Potential that exhibit unprecedented accuracy and sample efficiency and outperform invariant potentials with up to 1000x fewer reference data. I will discuss applications to a diverse set of materials systems, including Li diffusion, amorphous structures, heterogeneous catalysis, and water.
This is a Zoom seminar.
Free